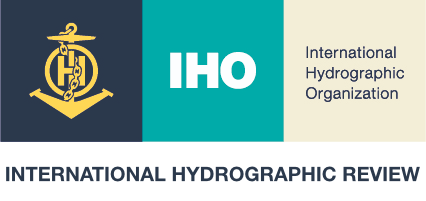
Automatic detection and classification of point clouds is a research topic of wide interest, as manual annotation of individual points is time consuming and inefficient for large surveys. This also holds for the emerging field of submerged vegetation detection, surveyed by bathymetric LiDAR. In the point clouds generated, current best practices perform sub-optimally in extracting vegetation data. To date, only a modest number of methodologies have been proposed to overcome this problem and furthermore only briefly discuss their findings in an extensive evaluation with annotated data. In contrast to the domain of sonar, where the practice of feature selection and comparison to annotated data has been well established over the last decades. This study proposes a similar methodology based on high-dimensional data analysis and clustering that is commonly deployed in other fields of research. In addition to the method, two datasets are presented for a detailed comparison to manually annotated data, in which the method performed at a mean precision score of 0.70 to 0.86, for all manual annotations. This demonstrates that the method is able to detect aquatic vegetation based on its structural characteristics of the bathymetric LiDAR point cloud, yielding results that are comparable to those obtained through manual annotation. In conclusion, the method presents an alternative workflow to current best practices and improves automated vegetation detection through the application of high dimensional data analysis.